Dataset
Remotely collected smartphone and smartwatch sensor data was obtained from the GSK study title: Novel Digital Technologies for the Assessment of Objective Measures and Patient Reported Outcomes in Rheumatoid Arthritis Patients: A Pilot Study Using a Wrist-Worn Device and Bespoke Mobile App. (212295, weaRAble-PRO)26. This observational study followed 30 participants diagnosed with moderate-to-severe RA and 30 matched HCs over 14 days. The population demographics, in-clinic, and relevant patient self-reported outcomes, as assessed at baseline, are reported in Table 1. RA participants were denoted as displaying moderate disability, RA (mod), or severe disability, RA (sev), as determined by their baseline RAPID-3 score. Note: Two RA participants withdrew immediately after enroling in the study. Data from these participants were not collected, leaving 28 RA participants, 28 matched HCs, and 2 unmatched HCs for a total of 58 participants. All study information, informed consent, study questions and instructions for conducting the guided tests were first drafted in the form of a survey instrument. The survey instrument was then programmed into the mobile app. All documentation including the study protocol, any amendments, and informed consent procedures, were reviewed and approved by Reliant Medical Group’s IRB. All participants provided written informed consent before any study procedures were undertaken. The study was conducted in accordance with the International Committee for Harmonisation principles of Good Clinical Practice and the Declaration of Helsinki. We refer the reader to Hamy et al.26 for further study details. In addition, participant requirement and data collection are outlined in the accompanying Supplementary Methods material.
Sensor-based data collection
The Apple Watch and iPhone were used to collect high frequency raw sensor data from predefined, (active) guided tests on a daily basis. Participants were prescribed daily to perform five iPhone-based assessments: WRT, a wrist range of motion (ROM) exercise12; WLK, a 30-second walking exercise12; PEG, a digital 9-hole peg test34; STS, a sit-to-stand transition exercise31,35; and LTS, a lie-to-stand transition exercise31,35. A brief overview of the guided tests prescribed in weaRAble-PRO are presented in Supplementary Table 8. In addition, the Apple Watch was used to continuously collect background sensor data (denoted passive data), as the participants went about their daily activities. Participants were asked to maintain a charge on both the Apple Watch and the iPhone, so that interruptions to monitoring and data transfer were kept to a minimum. Since night-time activity was also monitored, while participants were asleep, it was requested that charging should be done during the day, in a way that fit the participants’ schedules (e.g., charging in the morning while getting ready for the day). For more details on the activity monitoring features, see Supplementary Table 9.
Patient-reported outcomes
Patient-reported outcomes (PRO), most often self-report questionnaires, were administered to assess disease activity, symptoms, and health status and quality of life from the patients’ perspective36,37. The weaRAble-PRO study administered a selection of validated PRO measures for RA in complement to bespoke digital PRO assessments—that are validated in clinical trials, where the questions, response options, and the general approach to assessment were standardised for all participants. PROs were recorded on days 1, 7, and 14 of data collection. The PRO assessments administered to participants are outlined in Supplementary Table 7.
Smartwatch-based estimation of daily life patterns
In order to generate unobtrusive measures characterising physical activity and sleep in RA participants during daily life, the raw Apple Watch actigraphy (i.e., accelerometer) sensor data was transformed through a human activity recognition (HAR) sensor processing and deep convolutional neural network (DCNN) pipeline. Figure 7 illustrates how a deep convolutional neural network (DCNN) can transform raw Apple smartwatch sensor data to estimate a participant’s daily activity patterns in the weaRAble-PRO study using self-supervised learning (SSL). The construction of this pipeline yielded unobtrusively measured summary features of physical activity and sleep for RA participants, computed daily during normal life.
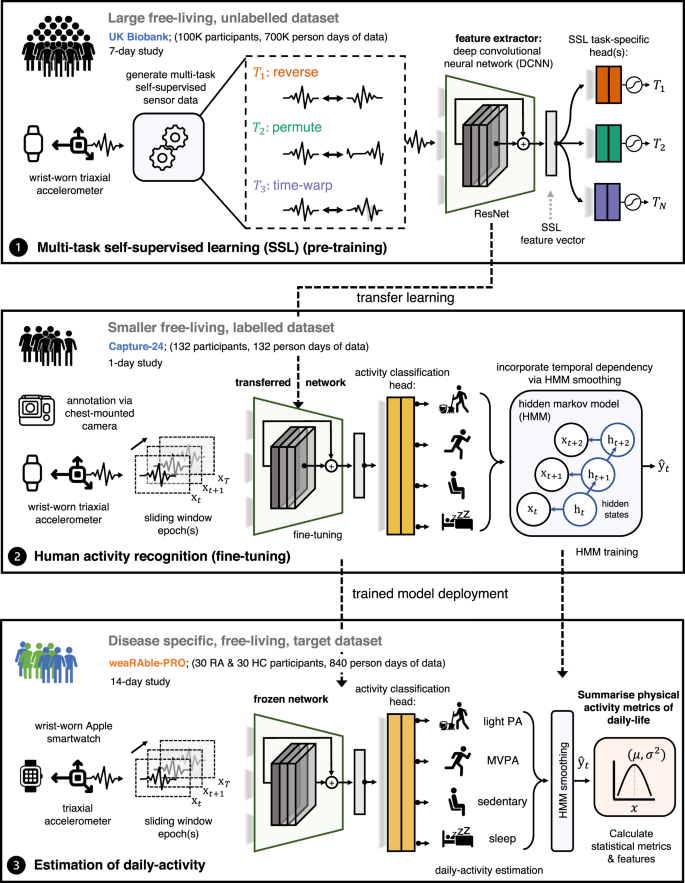
Continuous (passive) actigraphy was recorded from patients’ Apple smartwatch over the study duration. Deep convolutional neural networks (DCNN) were pre-trained on 700,000 person days in the publicly available UK Biobank using self-supervised learning—and fine-tuned with the Capture-24 dataset—to estimate participant’s daily activity patterns in the weaRAble-PRO study. Physical activity (PA) metrics of daily-life, for example, the time spent walking, the frequency of exercise, or the length and quality of sleep were investigated as markers to characterise symptoms of disease in people with RA compared to HC.
A deep convolutional neural network (DCNN) with a ResNet-V2 architecture was first pre-trained following a multi-task self-supervised learning (SSL) methodology on 100,000 participants, each participant contributing 7 days yielding roughly 700,000 person days of data, in the open-source UK biobank27. The SSL pre-trained model was then fine-tuned to perform activity recognition as a downstream task in the Capture-24 dataset.
The Capture-24 study is a manually labelled, free-living dataset—that is reflective of real-world environments—and is available for training an activity recognition model to be applied to the weaRAble-PRO study. In Capture-24, actigraphy data was collected for 24-h from 132 healthy volunteer participants with a Axivity AX3 wrist-worn device as they went their normal day. Activity labels provided by photographs automatically captured roughly every 30 seconds by a wearable camera for each participant. Capture-24 was labelled with 213 activity labels, standardised from the compendium of physical activities29. Activity labels were then summarised into a small number of free-living behaviour labels, defining activity classes in Capture-24.
There are two major labelling conventions used within Capture-24 that the model was trained to predict, defined as broad activity: {sleep, sedentary, light physical activity, moderate-to-vigorous physical activity (MVPA)}29,30; and fine-grained activity: {sleep, sitting/standing, mixed, vehicle, walking, bicycling}28.
HAR model predictions are essentially independent—meaning that the sequence of activities over each 30 s epoch incorporates no temporal information epoch-to-epoch, for instance how the previous epoch prediction affects the current, or next, activity prediction. In order to add temporal dependency to the “DCNN (SSL)” model, a Hidden Markov Model (HMM) was implemented in a post-processing step to obtain a more accurate sequence of predicted activities over the continuous 14-day data collection period as per Willetts, et al.28.
This Capture-24 fine-tuned “DCNN (SSL) + HMM” model was then implemented to estimate daily activities in weaRAble-PRO study data. For additional information of the HAR deep network, SSL, and other related information, we refer the reader to our previous work27. Further results relating to the “DCNN (SSL)” models are outlined in the Supplementary Table 1. The sensor processing pipeline developed for the Apple Watch in the weaRAble-PRO study is outlined in Supplementary Fig. 5 and within the accompanying Supplementary Methods.
Extraction of sensor-based outcomes
Wearable sensor-based features were derived from the smartphone during the active guided tasks and passively from the smartwatch during daily life. “Active” features, extracted from smartphone sensor-based measurements during the prescribed guided tests, aimed to capture specific aspects of RA physical function, related to pain, dexterity, mobility and fatigue12. In addition “passive” features were extracted from smartwatch sensor-based measurements, collected continuously in the background over the 14-day period. Daily activity predictions from the ML SSL model were summarised into general features measuring activity levels, period, duration and type of activity, as well as sleep detection and sleeping patterns. Furthermore, devised under the guidance of Rheumatologists, additional activity monitoring features specifically aimed at characterising well-known RA symptoms were also developed, such as morning stiffness and night-time restlessness.
The Supplementary Methods also detail algorithms used to extract active and passive features in the weaRAble-PRO study. For a full list of extracted sensor-based features in weaRAble-PRO, we refer the reader to Supplementary Table 9.
Statistical analysis
Univariate testing
Pair-wise differences groups between groups, for example HC vs. RA, or RA (mod) vs. RA (sev) were analysed for the equality in population median using the non-parametric Mann-Whitney U test (MWUT)38,39,40. One-way analysis of variance (ANOVA) tests were also used to assess differences between medians of multiple groups, for example HC vs. RA (mod) vs. RA (sev) were assessed using the Kruskal-Wallis (KWt) test by ranks41. The Brown-Forsythe (BF) test by (absolute deviation) of medians was used to investigate if various groups of data have been drawn with equal variances42.
Correlation analysis
Correlation analysis was utilised to determine the association or dependence between sets of random variables, such as the dependence between features, or to assess a features’ clinical utility by measuring the association to an established clinical metric. This study investigated the (linear) Pearson’s r correlation and the (non-linear) Spearman’s Rho ρ correlation between features, between features and PROs, and between clinical assessments to determine levels of association. The strengths of the correlations were classified as good-to-excellent (r > 0.75), moderate-to-good (r = 0.50–0.75), fair (r = 0.25–0.49) or no correlation (r < 0.25)43.
Feature reliability
Intra-rater (i.e., test-retest) reliability was determined using intra-class correlation coefficient (ICC) values44, which were used to assess the degree of similarity between repeated features over the course of the study for each patient. In this work, the ICC(3, k) was calculated45–which considers the two-way random average measures with k repeated measurements—for the 14-day session across subjects, where the raters k are the study days. Reliability was categorised as either poor (ICC < 0.5), moderate (ICC=0.5–0.75), good (ICC=0.75–0.9), or excellent (ICC > 0.9)46.
Correcting for multiple hypothesis testing
Multiple hypothesis testing was performed due to the large volume of features by controlling the false discovery rate (FDR) at level α using the linear step-up procedure introduced by Benjamini and Hochberg (BH)47,48.
Machine-learning estimation of RA status and severity
This work explored how state-of-the art machine learning (ML) models characterise the impact of RA during the daily life of participants in the 14-day weaRAble-PRO study. Multivariate modelling aimed to explore the ability of active, passive, and PRO measures to (1) distinguish RA participants from healthy controls (HC), and (2) to estimate RA disease severity: between RA participants with moderate symptoms (RA mod) and severe symptoms (RA sev) as binary classification tasks. Expansions of this analysis subsequently investigated how the in-clinic RAPID-3 assessment, a continuous measure of RA severity, could be estimated from the combination of PRO and sensor-based outcomes.
Overview of models
This analysis compared both linear and non-linear ML models to transform PRO and sensor-based outcomes to capture RA status and severity. Regularised linear regression (LR) models, with combinations of ℓ1 and ℓ2 priors, such as LR-lasso (ℓ1), LR-ridge (ℓ2), and LR-elastic-net (ℓ1 +ℓ2) were compared to yield predictive, yet sparse model solutions49. Further regularisation extensions were also investigated using the sparse-group lasso (SG-lasso)—an extension of the lasso that promotes both group sparsity and within group parameter-wise (ℓ2) sparsity, through a group lasso penalty and the lasso penalty—which aims to yield a sparse set of groups and also a sparse set of covariates in each selected group50,51.
Linear regression regularised models were also compared to decision tree (DT) based non-linear models, for instance the off-the-shelf Random Forest (RF)52 and Extreme Gradient Boosted Trees (XGB)53. Both LR- and DT-based models can intrinsically perform regression or classification depending on the task required. In the LR case, classification is denoted as logistic regression (though a logit-link function). NOTE: in this analysis LR can refer to both linear regression for continuous outputs or logistic regression for classification outputs. In the DT case, the mean prediction of the individual trees creates a continuous output for regression. For further details on the models employed in this study, we refer the reader to the Supplementary Methods.
Model evaluation
To determine the generalisability of our models, a stratified subject-wise k-fold cross-validation (CV) was employed. This consisted of randomly partitioning the dataset into k=5 folds, which was stratified with equal class proportions where possible. Participant data remained independent between training, validation, and testing splits. One set was denoted the training set (in-sample), and the remaining 20% of the dataset was then denoted testing set (out-of-sample) on which predictions were made.
Feature-wise and prediction-wise aggregation
In this work, we experimented with feature-wise and prediction-wise aggregation. In feature-wise aggregation, features were computed either as: daily feature values over the 14-day study period; the average daily feature value over a 7-day period (weekly); the average daily feature value over a 14-day period (fortnightly). Predictions could then be evaluated for each day (denoted observation-wise) or aggregated over all days through majority voting each individual prediction per subject (denoted subject-wise). For example, daily and weekly averaged features result in daily, or weekly predictions (i.e., observation-wise), which were summarised into subject-wise outcomes by majority voting over the repeated predictions.
Evaluation metrics
Multi-class classification metrics were reported as the observation-wise median and interquartile (IQR) range over one CV, as well as the subject-wise outcome for that CV, using: auroc, area under the receiver operating characteristic curve; k, Cohen’s kappa statistic54,55; F1, F1-score. The coefficient of determination, r2, the mean absolute error (MAE), and root-mean squared error (RMSE) were used to evaluate modelling the (continuous) in-clinic RAPID-3 scores56.